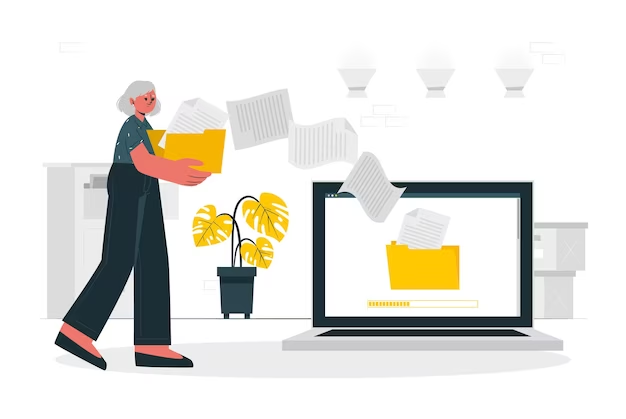
Maintaining clean and accurate data is of utmost importance. Poor-quality data can lead to costly errors, inefficiencies, and missed opportunities. Open source data cleansing tools offer a cost-effective and efficient way to clean, transform, and enhance your data. In this comprehensive guide, we will explore the world of open source data cleansing tools and their significance and provide a list of the 11 best tools to help you make informed decisions.
What Is Data Cleansing?
Data cleansing, also known as data scrubbing or data cleaning, is identifying and correcting errors, inconsistencies, and inaccuracies in datasets. This crucial step involves detecting and rectifying duplicate records, missing values, and other anomalies adversely affecting data quality. Ensuring your data is accurate, complete, and consistent can enhance decision-making, improve operational efficiency, and gain a competitive edge.
Why Is Data Cleansing Important?
Effective data cleansing is essential for several reasons:
- Improved Data Quality: Clean data ensures that your business decisions are based on accurate and reliable information.
- Enhanced Productivity: Clean data streamlines processes and reduces the time spent on manual data correction.
- Better Customer Experiences: Accurate customer data leads to personalized and more effective marketing campaigns.
- Regulatory Compliance: Many industries have regulations that require data accuracy and privacy.
What Are Open Source Data Cleansing Tools?
Open source data cleansing tools are software solutions that allow organizations to clean and enhance their data without expensive proprietary software. These tools are typically developed by a community of contributors, making them cost-effective and accessible. They provide a wide range of data cleaning, transformation, and enrichment functionalities, making them invaluable for businesses of all sizes.
11 Best Open Source Data Cleansing Tools
- Open Source Data Cleansing Tools #1 OpenRefine
- Open Source Data Cleansing Tools #2 Talend
- Open Source Data Cleansing Tools #3 DataWrangler
- Open Source Data Cleansing Tools #4 Trifacta
- Open Source Data Cleansing Tools #5 Apache Nifi
- Open Source Data Cleansing Tools #6 Google Refine
- Open Source Data Cleansing Tools #7 DataCleaner
- Open Source Data Cleansing Tools #8 Dataddo
- Open Source Data Cleansing Tools #9 Kettle (Pentaho Data Integration)
- Open Source Data Cleansing Tools #10 Data Ladder
- Open Source Data Cleansing Tools #11 Apache Tika
Let’s dive into the world of open source data cleansing tools. Here are 11 top options:
#1. OpenRefine
OpenRefine is a powerful and user-friendly open-source tool that offers data cleaning and transformation capabilities. It excels at handling messy data and supports various data formats.
Features:
- Data Exploration: Visualize and understand your data through facets and charts.
- Data Transformation: Easily transform data using a user-friendly interface.
- Data Reconciliation: Match data against external data sources for accuracy.
- Extensible: Extend functionality through plugins and scripting.
Pros:
- Intuitive and user-friendly.
- Great for cleaning and standardizing messy data.
- Supports a wide range of data formats.
Cons:
- Limited support for large datasets.
- Some advanced transformations may require scripting.
#2. Talend
Talend is a popular open-source data integration tool with robust data cleansing features. It offers a comprehensive suite for data integration, data quality, and data governance.
Features:
- Data Integration: Seamlessly integrate data from various sources.
- Data Quality: Cleanse and standardize data with built-in rules.
- Data Governance: Enforce data policies and compliance.
- Scalable: Suitable for small businesses and large enterprises.
Pros:
- Comprehensive data integration capabilities.
- Strong data quality and governance features.
- Scalable to meet the needs of large organizations.
Cons:
- It may have a steeper learning curve for beginners.
- Advanced features may require premium versions.
#3. DataWrangler
DataWrangler is an accessible and user-friendly data cleansing tool developed by Stanford University. It allows users to interactively clean and transform data without writing code.
Features:
- Interactive Data Cleaning: Clean and transform data through a visual interface.
- Automatic Suggestions: DataWrangler suggests transformations for common data issues.
- Export Options: Export cleaned data to various formats.
- Community Support: Access to user-contributed transformations.
Pros:
- Extremely user-friendly, no coding required.
- Offers intelligent data transformation suggestions.
- Suitable for users with little technical expertise.
Cons:
- Limited advanced data cleansing features.
- It may not be suitable for vast datasets.
#4. Trifacta
Trifacta is a cloud-based data preparation platform that offers data cleansing, structuring, and enrichment capabilities. It’s designed for data professionals and business users alike.
Features:
- Data Wrangling: Easily clean and structured data with a visual interface.
- Intelligent Suggestions: Trifacta suggests data transformations based on patterns.
- Collaboration: Collaborate with team members on data preparation projects.
- Scalable: Suitable for enterprises with large datasets.
Pros:
- Powerful data wrangling capabilities.
- Intelligent data transformation suggestions.
- Collaborative features for teams.
Cons:
- Cloud-based may not be suitable for organizations with strict data security requirements.
- Pricing can be on the higher side for large enterprises.
#5. Apache NiFi
Apache NiFi is an open source data integration tool that provides data cleansing, transformation, and routing capabilities. It is designed for data flow automation and management.
Features:
- Data Ingestion: Easily ingest data from various sources.
- Data Transformation: Cleanse and transform data on the fly.
- Data Routing: Route data to the appropriate destinations.
- Scalable: Suitable for real-time data processing.
Pros:
- Excellent for data flow automation and management.
- Supports real-time data processing.
- Scalable for handling large data volumes.
Cons:
- It may require some technical expertise to set up and configure.
- Limited data quality features compared to specialized tools.
#6. Google Refine (formerly Freebase Gridworks)
Google Refine, previously known as Freebase Gridworks, is a free, open-source tool for cleaning and transforming data. It offers a user-friendly interface and integrates well with other Google products.
Features:
- Data Transformation: Clean and transform data interactively.
- Faceted Browsing: Explore data using facets and filters.
- Integration: Seamlessly integrates with Google Sheets and other Google tools.
- Open Source: Continues to have an active open source community.
Pros:
- User-friendly and intuitive interface.
- Integration with Google products.
- Active open source community.
Cons:
- Limited support for large datasets.
- Google has shifted focus away from the project, so community support is crucial.
#7. DataCleaner
DataCleaner is an open-source data quality solution that provides data profiling, cleansing, and enrichment capabilities. It helps organizations maintain clean and accurate data.
Features:
- Data Profiling: Analyze and profile data for quality issues.
- Data Cleansing: Cleanse data using predefined and custom rules.
- Data Enrichment: Enhance data with external sources.
- Integration: Integrates with various data sources and platforms.
Pros:
- Comprehensive data profiling and cleansing capabilities.
- Support for data enrichment.
- Integrates with a variety of data sources.
Cons:
- The user interface may not be as intuitive as some other tools.
- Limited community support compared to larger projects.
#8. Dataddo
Dataddo is an open source data integration and ETL (Extract, Transform, Load) platform that offers data cleansing features. It simplifies the process of collecting and transforming data from various sources.
Features:
- Data Collection: Collect data from multiple sources, including APIs.
- Data Transformation: Cleanse and transform data using a visual interface.
- Data Scheduling: Automate data collection and transformation tasks.
- Scalable: Suitable for businesses of all sizes.
Pros:
- Simplifies data integration and transformation.
- Supports a wide range of data sources.
- Offers automation and scheduling options.
Cons:
- It may require some learning for users new to ETL concepts.
- Limited advanced data cleansing features.
#9. Kettle (Pentaho Data Integration)
Kettle, or Pentaho Data Integration, is a robust open-source ETL tool with data cleansing and transformation capabilities. It’s part of the Pentaho suite.
Features:
- ETL Functionality: Extract, transform, and load data from various sources.
- Data Cleansing: Cleanse and standardize data with built-in transformations.
- Workflow Orchestration: Design complex data workflows.
- Scalable: Suitable for enterprise-level data integration.
Pros:
- Comprehensive ETL capabilities.
- Vital data cleansing and transformation features.
- Suitable for large-scale data integration projects.
Cons:
- Learning curve for beginners.
- Community support may be limited compared to paid options.
#10. Data Ladder
Data Ladder is an open-source data quality tool specializing in data cleansing, deduplication, and standardization. It helps organizations maintain clean and accurate data.
Features:
- Data Profiling: Profile data to identify quality issues.
- Data Cleansing: Cleanse data using customizable rules.
- Deduplication: Identify and remove duplicate records.
- Integration: Integrates with various data sources.
Pros:
- Strong focus on data cleansing and deduplication.
- Customizable data cleansing rules.
- Integration with popular data sources.
Cons:
- The user interface may not be as intuitive as some other tools.
- Limited advanced data quality features.
#11. Apache Tika
Apache Tika is an open-source content analysis and data extraction tool. While not solely a data cleansing tool, it can extract structured data from unstructured sources, which can be crucial to data preparation.
Features:
- Content Analysis: Extract text and metadata from various file formats.
- Structured Data Extraction: Extract structured data from unstructured sources.
- Extensible: Supports plugins for additional functionality.
- Integration: Integrates with other Apache projects.
Pros:
- Useful for extracting structured data from unstructured sources.
- Part of the Apache ecosystem.
- Extensible through plugins.
Cons:
- It may not offer the full range of data cleansing features found in specialized tools.
- Requires technical expertise to configure and use effectively.
How to Choose the Best Open-Source Data Cleansing Tool?
Now that we’ve explored these 11 open source data cleansing tools, you may be wondering how to choose the best one for your needs. Here are some factors to consider:
- Data Complexity: Assess the complexity of your data and choose a tool that can handle it effectively.
- User-Friendliness: Consider the technical expertise of your team and select a tool that matches their skill level.
- Scalability: Ensure the tool can grow with your data needs.
- Integration: Check if the tool integrates smoothly with your existing data ecosystem.
- Community Support: Look for active communities and user support forums.
- Budget: Evaluate your budget and the cost of implementing and maintaining the tool.
Pros and Cons of Open Source Data Cleansing Tools
Open Source Data Cleansing Tools offer a range of benefits and drawbacks for users seeking to clean and enhance their data. Here are some of the advantages and disadvantages of using these tools:
Pros:
- Cost-Efficiency: Open Source Data Cleansing Tools are typically free, making them a cost-effective option for organizations and individuals looking to improve data quality without significant expenses.
- Customization: These tools often provide a high degree of customization, allowing users to tailor data cleansing processes to their specific needs and data types.
- Community Collaboration: Many open-source projects related to data cleansing have active communities of developers and users who collaborate to enhance tool functionality, share best practices, and troubleshoot issues.
- Transparency: Open Source Data Cleansing Tools are transparent in their source code, enabling users to inspect and verify data cleansing procedures, which can instill trust and security in the data cleaning process.
- No Vendor Lock-In: There is no vendor lock-in with open-source tools, offering users freedom and independence in choosing and maintaining their data cleansing solutions.
- Continuous Improvement: Open-source data cleansing projects often benefit from ongoing contributions and updates from the community, ensuring that the tools stay current with evolving data challenges.
Cons:
- Complexity: Some Open Source Data Cleansing Tools can be complex to set up and use, requiring a certain level of technical expertise, which may be a barrier for non-technical users.
- Limited Documentation: While many open-source data cleansing projects provide comprehensive documentation, some may lack detailed guides, potentially making it challenging for beginners to start using them effectively.
- Support Variability: Although there is often a community of users willing to provide support, the quality and responsiveness of this support can vary, and users may not receive the same level of support as with paid solutions.
- Maintenance Burden: Users of Open Source Data Cleansing Tools are responsible for maintaining and updating the software, which can be burdensome for those with limited technical resources or expertise.
- Security Risks: Open source exposes Open Source Data Cleansing Tools to potential security vulnerabilities if not regularly updated and patched, requiring diligent monitoring and maintenance.
- Integration Challenges: Some Open Source Data Cleansing Tools may not have built-in integrations with other software and systems, potentially necessitating additional development efforts for seamless integration into existing workflows.
In conclusion, Open Source Data Cleansing Tools offer many advantages, including cost-efficiency, customization, and community collaboration. However, they also present complexity, support, and maintenance challenges. Whether to use Open Source Data Cleansing Tools or proprietary solutions depends on your specific data cleansing needs, available resources, and technical capabilities. Careful evaluation of these factors is essential before making a decision.
Conclusion
Data cleansing is essential in ensuring that your data is accurate, reliable, and valuable. Open source data cleansing tools provide cost-effective solutions for organizations of all sizes. Whether you choose a user-friendly option like OpenRefine or a more comprehensive platform like Talend, the right tool can help you maintain data integrity and make informed decisions.
By considering your specific requirements, budget, and the complexity of your data, you can select the best open-source data cleansing tool to meet your organization’s needs. Clean data is the foundation of successful data-driven decision-making, and these tools can help you achieve that goal efficiently and effectively.